Presenter Profile
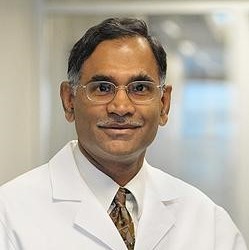
Rohit P. Shenoi, MD
Attending Physician, Emergency Center
Texas Children’s Hospital
rshenoi@bcm.edu
Rohit P. Shenoi is a Professor of Pediatrics at Baylor College of Medicine and an Attending Physician in the Emergency Center at Texas Children’s Hospital. Dr. Shenoi has a long-standing interest in injury prevention, specifically - drowning, opioid stewardship, and screening youth for suicide and drugs in the Emergency Department
Presentations
Economic Burden of Pediatric Drowning in Texas
Rohit P. Shenoi, MD
Linh Nguyen, PhD.
Drowning is the leading cause of unintentional injury death in US children aged 1-4 years. Texas led the US in fatal pediatric drownings between 2018-2021. We assessed the economic burden of unintentional fatal and non-fatal pediatric drowning in Texas.
This was a cross-sectional study involving children aged 0-17 years treated for unintentional drowning in Texas hospitals during 2016-2022. We obtained inpatient (IP) and outpatient (OP) data from the Texas Health Care Information Collection. We selected fatal and non-fatal unintentional drowning (including water-transport related) encounters and excluded undetermined intent drowning, subsequent and sequela encounters. Data included demographics, diagnoses, procedure codes, body of water, length of stay, discharge status, and facility charges. The economic burden comprised direct medical costs (DMC) and indirect costs (IC) associated with drowning IP and OP treatment. DMC includes facility and professional costs. Facility costs (accommodation, ancillary services, outpatient charges, ambulance fees) were estimated by multiplying the facility charges by mean year-specific, county-specific cost-to-charge ratio. Professional costs were the product of facility costs and professional fee ratio for drowning. DMC was converted to 2022 US$ using the Consumer Price Index for All Urban Consumers Medical Care Services. To estimate IC, we measured the caregiver productivity loss based on time needed to care for a hospitalized patient or to visit the emergency department (ED). Workdays lost (#inpatient days or 1 day for ED visit) were multiplied by the average daily compensation for full-time employees and the average wage multiplier incurred by the employer due to worker absence to compute economic loss of productivity. We performed descriptive analysis and utilized non-parametric tests to estimate the economic burden and cost variations among groups.
There were 1,339 pediatric hospitalizations in Texas during 2016-2022. Most drownings occurred in swimming pools (41%); Fatal drownings occurred in 15% of hospitalizations. Most hospitalizations occurred in children aged 1-4-years (64%). Males, non-Hispanic White children, and commercially-insured constituted the majority (63%,39% and 52%, respectively) of drowning patients.
DMC IP costs averaged $62,398 with no significant differences in mean costs by sex, race/ethnicity, or insurance. Fatal drownings incurred significantly higher mean IP costs ($99,248) than non-fatal drowning ($55,852)(p<0.001). Watercraft-related drownings ($121,393) and bathtub drownings ($107,070)(p<0.001) had the highest mean IP costs. Mean DMC OP costs were $2,593 and varied by race/ethnicity, insurance, and body of water (p<0.001). Watercraft-related drownings incurred the highest mean OP costs ($3,324). The mean productivity loss was 6.2 days. IC averaged $3,299 and varied by age, race/ethnicity, insurance, discharge status, and water body (p<0.05). Non-fatal drownings had higher mean IC ($3,356) than fatal drownings ($2,974). Watercraft-related drownings had the highest mean IC ($8,707), followed by bathtub drownings ($4,982)(p<0.001).
Pediatric drowning is a significant economic burden in Texas. Costs varied by age, discharge status, and body of water. Fatal IP and watercraft-related drownings incurred the highest direct and indirect costs.
1. Compute direct and indirect costs from drowning injuries
2. Discuss the economic burden of pediatric drowning in Texas.
3. Recognize groups that incur the highest drowning-related costs
Studying the epidemiology of drowning from media reports using artificial intelligence
Aedan Villegas, BS
Shaila Zaman, PhD
Rohit P. Shenoi, MD
Media reports are a source of information on fatal drowning. The narratives provide information about demographics and contextual information of victims. We sought to describe the epidemiology of fatal drowning in the metropolitan Houston region using artificial intelligence to analyze media report narratives.
This was a cross-sectional study of victims of unintentional drownings of all ages who drowned in the 8-county metropolitan Houston region from 2016-2022. We queried the search platform – NexisUni using keywords “drown” and “submersion” to access online media archives of fatal drownings from the following formats: print; blog, video, and audio records. Temporal data, demographic information (age, sex, ethnicity, race), risk and protective factors were obtained from the narratives. We developed an automated method to extract the information using the natural language processing (NLP) capabilities of OpenAI's GPT-3.5 model. The OpenAI API significantly enhances the efficiency and accuracy of extracting structured information from unstructured text by implementing advanced NLP techniques, facilitating efficient handling of large datasets with precision that surpasses manual methods. Using OpenAI API, we focused on extracting key factors such as swimming ability, supervision status, alcohol use, and life vest use from narrative descriptions of drowning circumstances. Our approach involved prompt engineering that guided the model to output structured responses in a dictionary format, ensuring consistency in the extracted data. The method was implemented in Python, where we utilized the OpenAI API to process each narrative in our dataset. The responses were then parsed and organized into a structured format suitable for further analysis. We used descriptive statistics for reporting results.
There were 133 media reports of drowning between 2016-2022 in metropolitan Houston. We analyzed 110 cases of drowning after excluding non-fatal (n=16), homicides (n=4) and those external to the Houston region (n=3). The mean age of drowning victims was 25.5 years (Std. Dev. ±23). There were 49 (44.5%) children; males constituted 81% of reports. The majority of drownings occurred in natural water (73%). The rest occurred in confined water (swimming pools, bathtubs)(26%) and unknown (1%) Life jackets were used by 33% of drowning victims; their use was unknown in 67% of cases. Fourteen percent of drowning victims knew how to swim, whereas 19% did not know how to swim. Swimming ability was unknown in 67% of cases. A majority of drowning victims did not consume alcohol (65%). Only 1% consumed alcohol and its use was unknown in 34%. Among children, 65% were supervised, 10% were unsupervised. Supervision was unknown in 25%.
Media reports are an alternate source of data on fatal drowning. Artificial intelligence is a novel method to extract drowning information from media report narratives for epidemiological purposes. However, the utility of media reports in drowning surveillance and prevention is limited, given biases in reporting and sub-optimal reporting of information on protective and risk factors. Engagement with media outlets to include drowning prevention information during reporting is encouraged.
1. Explain how artificial intelligence can be used in injury surveillance
2. Discuss the epidemiology of drowning obtained from media reports.
3. Recognize the limitations of media reports in describing the epidemiology of drowning
Drowning Prevention
Rohit P. Shenoi, MD
Lorrie Lynn, MA, CPSTI